By: Faisal Awartani (Ph.D.)
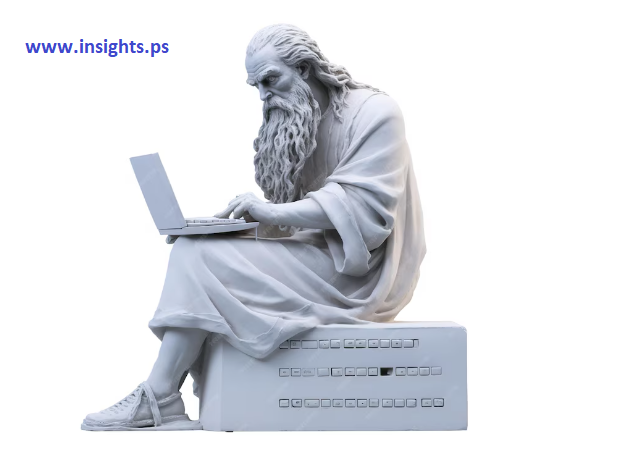
Introduction
In today’s fast-paced technological world, data science is driving innovations across industries. However, technical skills alone are no longer enough for data scientists to thrive. Thought leadership is increasingly essential, as it promotes forward-thinking, challenges conventional wisdom, and fosters new perspectives that push boundaries. Integrating philosophical thinking into data science could further enhance this thought leadership, helping data scientists generate transformative ideas that can evolve into meaningful data products.
The Role of Thought Leadership in Data Science
Thought leadership goes beyond mastering algorithms and statistical techniques; it involves guiding others with visionary insights, challenging the status quo, and offering novel solutions to complex problems. In the realm of data science, this can mean identifying new patterns of thinking, spotting unseen connections within data, or envisioning future applications of data products before the market demands them.
Thought leaders inspire peers and organizations by anticipating trends, leading conversations, and translating insights into actionable outcomes. In an era where data is abundant, those who can interpret data in ways that bring new understanding or societal benefit will shape the future of this field.
Why Philosophy Matters for Data Scientists
At first glance, philosophy may seem like an unusual ally to data science, but it brings powerful tools that can help data scientists excel:
1. Critical Thinking and Logic: Philosophy trains the mind to think clearly and systematically. It cultivates the ability to analyze problems from multiple perspectives and question assumptions, which can enhance problem-solving in data science. This leads to more robust models and solutions.
2. Ethics and Data Responsibility: With the growing concerns around privacy, bias, and the ethical use of data, philosophical frameworks help data scientists navigate moral questions. Thought leaders in data science must not only generate ideas but also consider their societal implications.
3. Conceptual Thinking: Philosophy fosters abstract thinking and conceptual clarity. Data scientists dealing with ambiguous data sets or unstructured data can benefit from philosophical approaches to organizing thought, which can ultimately help structure data in innovative ways.
4. Creativity and Innovation: Philosophers are often tasked with imagining scenarios beyond what is currently possible. For data scientists, this imaginative capacity is critical when envisioning novel data products or discovering insights that others might miss.
Transforming Ideas into Data Products
For data scientists, thought leadership isn’t just about theoretical knowledge; it’s about turning innovative ideas into tangible products that solve real-world problems. Philosophy can serve as a catalyst for creative thinking, helping data scientists move beyond conventional approaches to conceive groundbreaking data products.
For example, a data scientist with a strong foundation in ethics may develop a new AI model that balances accuracy with fairness, while a professional with philosophical training in logic may discover new methods for pattern recognition in large data sets. Ultimately, philosophy can help data scientists ensure that their innovations are not only technically proficient but also socially valuable and forward-looking.
Conclusion In a world increasingly shaped by data, thought leadership in data science is essential for innovation. By drawing on the critical, ethical, and creative tools of philosophy, data scientists can elevate their performance, generating ideas that are not only insightful but also transformative. As the field continues to evolve, those who can blend technical expertise with philosophical depth will lead the way in creating data products that address both current and future challenges
Comments