Faisal Awartani (Ph.D.)
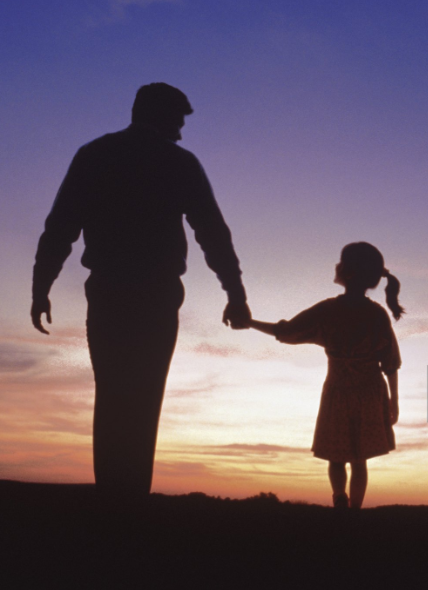
Schools and universities are facing a significant challenge in detecting text generated by artificial intelligence (AI). The detection of AI-generated text can only occur under specific circumstances, which are outlined below. Firstly, the request statement used to generate the research paper or article, which serves as the primary input for the model, must remain the same. Any variations in the request statement will result in different AI-generated text. This is because AI models are chaotic, meaning that even small changes in the input can result in significant changes in the output. Secondly, since AI models continually learn, any testing of a paper's authenticity by a professor or teacher will be affected by changes in the model's parameters. As a result, the same inputs for large language models at different times will produce different outputs. Lastly, the training data set for the model is continuously changing. This dataset serves as the "information" space the model searches through to find related topics to the request of interest. From a cybersecurity perspective, AI models for generating text can be compared to a dynamic virus that continuously changes itself. Therefore, it is difficult to develop a cybersecurity algorithm that can detect such viruses. Detecting AI-generated text is challenging from a conceptual viewpoint. It is possible to design software that can detect AI-generated articles if specific conditions are met. These conditions include using a fixed large language model to generate text, knowing the exact input statement used to generate the receptive article, and a fixed space used to search for relevant information to be fed to the model that doesn't change after the text is generated. However, achieving these requirements is nearly impossible. Therefore, developing systems to detect AI-generated text is unfeasible and will suffer from large Type I and Type II errors.
Top of Form
Comments